.jpg)
数据挖掘:作业 04
数据挖掘:实验四 Logistic回归与KNN算法
代码地址:Github
实验目的和要求
通过在Python中的实例应用,分析掌握KNN算法的基本原理,加深对KNN算法的理解,并掌握将算法应用于实际的方法、步骤。
实验内容和原理
- 在Python中完成多分类的Logistic回归模型的程序设计;
- 在Python中完成KNN算法模型的数据输入、参数设置;
- 对Python中KNN算法的实例数据输出结果进行分析。
操作方法和实验步骤
体验KNN算法。给定以下的疾病诊断数据集。这个数据集包含1个病人代号的字段、5个条件字段(喉咙痛、发烧、淋巴腺肿胀、充血、头痛)以及一个目标字段(诊断结果)
病人代号 | 喉咙痛 | 发烧 | 淋巴腺肿胀 | 充血 | 头痛 | 诊断结果 |
---|---|---|---|---|---|---|
1 | Yes | Yes | Yes | Yes | Yes | 链球菌喉炎 |
2 | No | No | No | Yes | Yes | 过敏 |
3 | Yes | Yes | No | Yes | No | 感冒 |
4 | Yes | No | Yes | No | No | 链球菌喉炎 |
5 | No | Yes | No | Yes | No | 感冒 |
6 | No | No | No | Yes | No | 过敏 |
7 | No | No | Yes | No | No | 链球菌喉炎 |
8 | Yes | No | No | Yes | Yes | 过敏 |
9 | No | Yes | No | Yes | Yes | 感冒 |
10 | Yes | Yes | No | Yes | Yes | 感冒 |
11 | NO | NO | YES | YES | YES | ? |
12 | YES | YES | NO | NO | YES | ? |
-
利用KNN预测11、12号病人的诊断结果 (K = 3)
Distance 的计算方式:
-
Distance (Yes, No) = 1
-
Distance (Yes, Yes) = 0
-
Distance (No, No) = 0
两客户的Distance为每一字段之Distance的加总,即Manhattan Distance / City Block Distance
-
下载数据集cancer_X.csv,cancer_y.csv,amazon_X.csv,amazon_y.csv,实现如下功能:
- 利用Python语言实现多分类的Logistic回归模型的程序设计,并给出上述两组数据的Logistic回归模型的准确率(测试集与训练集相同)。
- 利用Python语言实现KNN算法,并给出不同的邻近点个数k对应的预测准确率(测试集与训练集相同)。
实验结果和分析
Answer01
将疾病种类编码:
疾病种类 | 编码 |
---|---|
链球菌喉炎 | 0 |
过敏 | 1 |
感冒 | 2 |
def _square_distance(v1, v2):
sum = 0
for i in range(len(v1)):
if v1[i] != v2[i]:
sum += 1
return sum
def _vote(ys):
vote_dict = {}
for y in ys:
y = y[0]
if y not in vote_dict.keys():
vote_dict[y] = 1
else:
vote_dict[y] += 1
sorted_vote_dict = sorted(vote_dict.items(), key=operator.itemgetter(1), reverse=True)
return sorted_vote_dict[0][0] # 将已经记好的数进行一个自大而小的排列(则是一个降序排序),若reverse是false,则是自小而大.(升序排序)
class KNN:
def __init__(self, k=3):
self.x = None
self.y = None
self.k = k
# 用于后期的模型计算,输入训练集目标数据以及训练数据x。
def fit(self, x, y):
self.x = x
self.y = y
def predict(self, x):
y_pred = [] # 定义一个空集合,用于y_pred(预测)
# 进行遍历,得到x的长度,range是一个从一开始,直到x,步长为1
for i in range(len(x)):
dist_arr = [_square_distance(x[i], self.x[j]) for j in range(len(self.x))]
sorted_index = np.argsort(dist_arr)
top_k_index = sorted_index[:self.k]
y_pred.append(_vote(ys=self.y[top_k_index])) # 调用了_vote函数,属于自身调用,去进行分类
return np.array(y_pred)
def score(self, y_true=None, y_pred=None):
if y_true is None and y_pred is None:
y_pred = self.predict(self.x)
y_true = self.y
score = 0.0
for i in range(len(y_true)):
if y_true[i] == y_pred[i]:
score += 1
score /= len(y_true)
return score

Answer02
使用 sklearn.linear_model.LogisticRegression 进行多分类
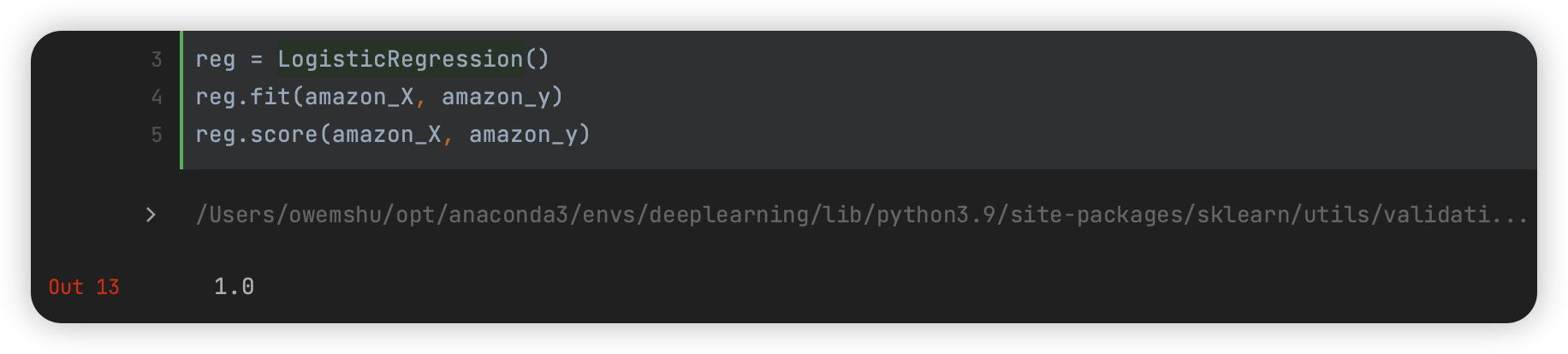
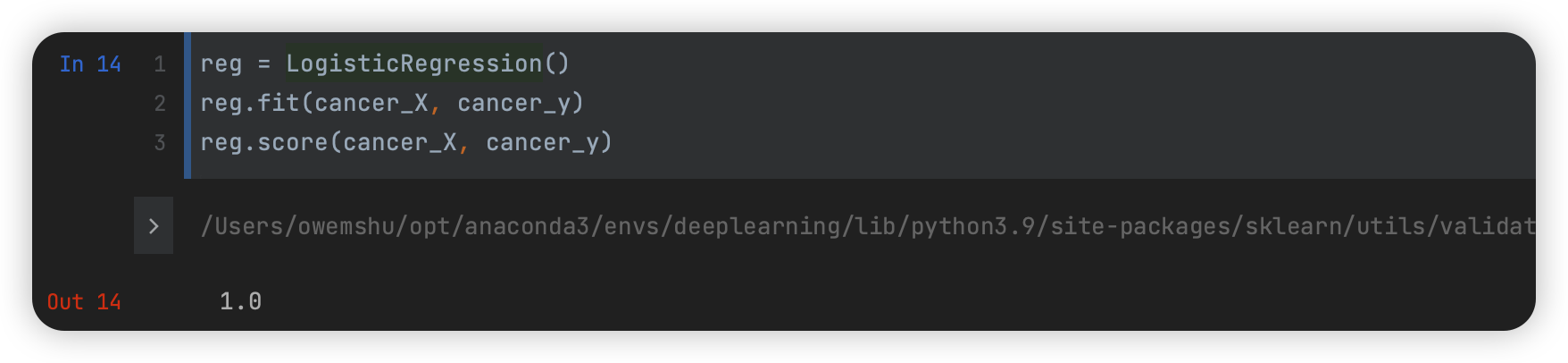
使用逻辑回归进行多分类
class SoftmaxRegression:
def __init__(self):
self.n_samples = None
self.n_features = None
self.n_classes = None
self.weights = None
self.all_loss = list()
def fit(self, x, y, iters=1000, alpha=0.1, lam=0.01):
self.n_samples, self.n_features = x.shape
self.n_classes = y.shape[1]
# self.weights = np.random.rand(self.n_classes, self.n_features) # 使用随机初始化会造成运算异常
self.weights = np.zeros((self.n_classes, self.n_features))
for i in range(iters):
probs = self._softmax(x)
loss = - (1 / self.n_samples) * np.sum(y * np.nan_to_num(np.log(probs)))
self.all_loss.append(loss)
dw = -(0.5 / self.n_samples) * np.dot((y - probs).T, x) + lam * self.weights
dw[:, 0] -= lam * self.weights[:, 0]
self.weights -= alpha * dw
def predict(self, test_x):
probs = self._softmax(test_x)
return np.argmax(probs, axis=1).reshape((-1, 1))
def score(self, test_x, test_y):
score = 0.0
for i in range(len(test_y)):
if (self.predict([test_x[i]]) == np.argmax(test_y[i])).any():
score += 1
return score / len(test_y)
def _softmax(self, x):
scores = np.dot(x, self.weights.T)
sm = np.exp(scores) / np.sum(np.exp(scores), axis=1, keepdims=True)
sm = np.nan_to_num(sm)
return sm
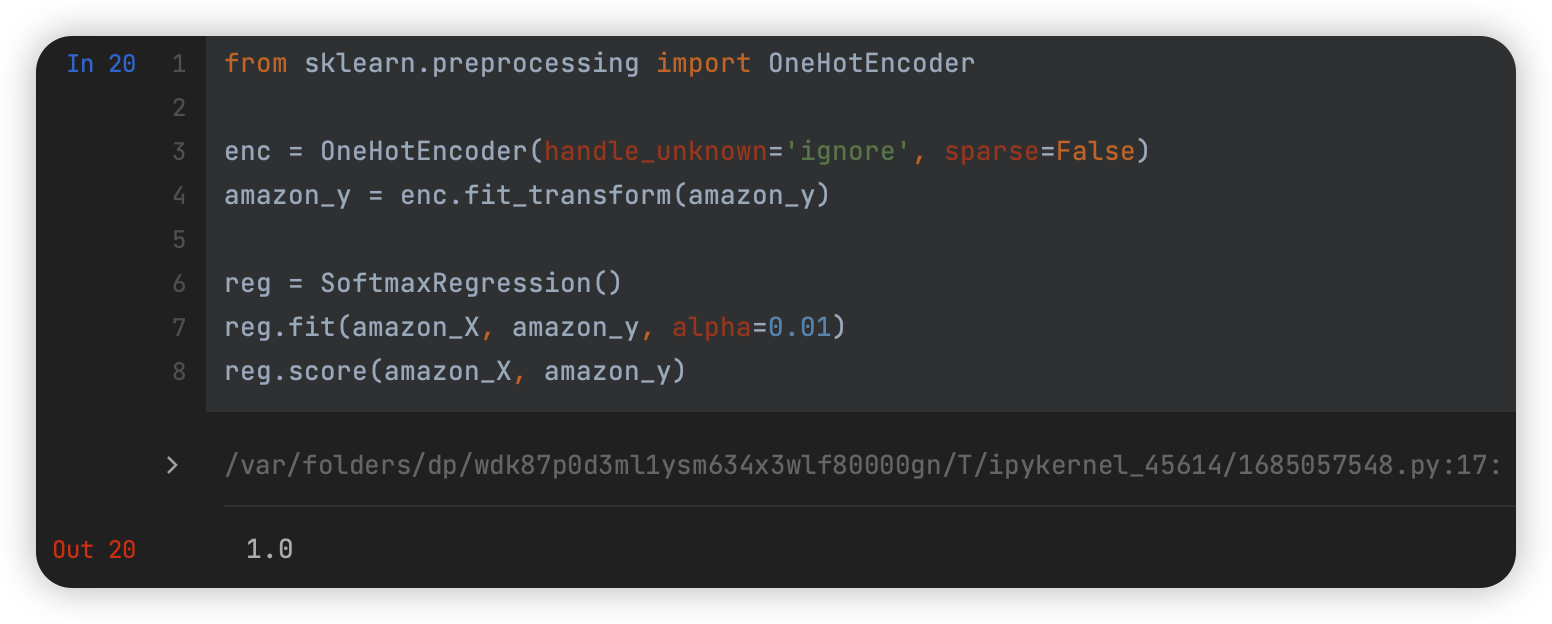
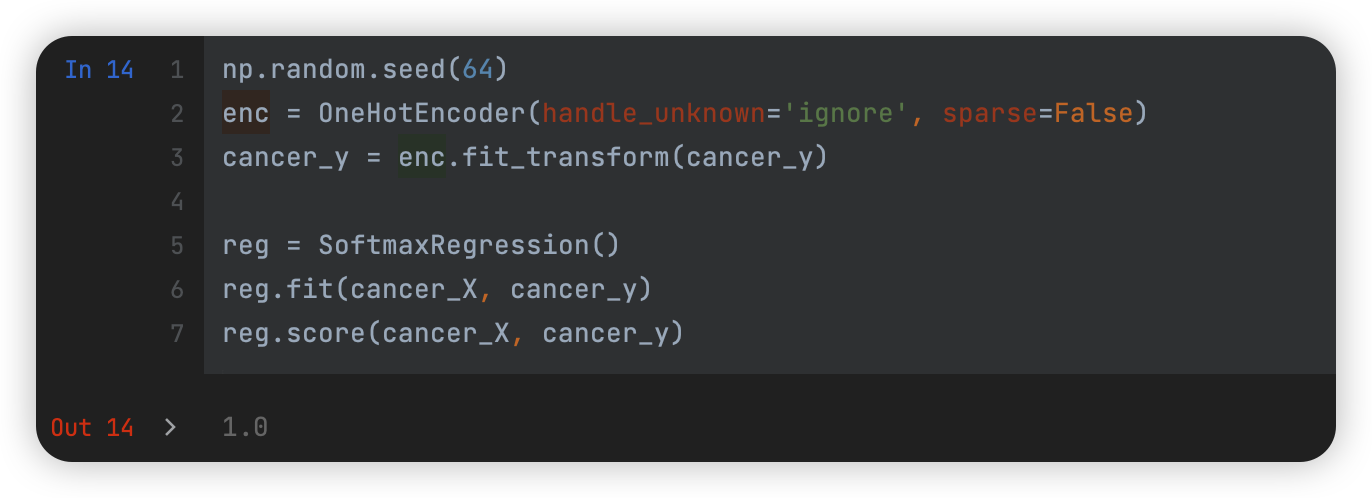
使用 KNN 进行多分类
代码同 Answer01


本文是原创文章,采用 CC BY-NC-ND 4.0 协议,完整转载请注明来自 Owen
评论
匿名评论
隐私政策
你无需删除空行,直接评论以获取最佳展示效果